I completed my Ph.D. in Computer Science from Cornell University, where I had the privilege of working with Prof. Karthik Sridharan and Prof. Robert D. Kleinberg. Before that, I completed my undergraduate studies in Computer Science and Engineering at the Indian Institute of Technology Kanpur (IIT Kanpur), where I was awarded the President’s Gold Medal. Between my time at IIT Kanpur and Cornell, I spent a year at Google Research as part of the inaugural Brain Residency Program .
Research Highlights and Selected Papers
-
Remember What You Want to Forget: Algorithms for Machine Unlearning,
with Jayadev Acharya, Gautam Kamath, and Ananda Theertha Suresh (NeurIPS 2021). -
Ticketed Learning-Unlearning Schemes,
with Badih Ghazi, Pritish Kamath, Ravi Kumar, Pasin Manurangsi, and Chiyuan Zhang (COLT 2023).
-
Selective Sampling and Imitation Learning via Online Regression,
with Karthik Sridharan, Wen Sun, and Runzhe Wu (NeurIPS 2023). -
On the Complexity of Adversarial Decision Making,
with Dylan J. Foster, Alexander Rakhlin and Karthik Sridharan. (NeurIPS 2022, oral presentation). -
Hybrid RL: Using Both Offline and Online Data Can Make RL Efficient,
with Yuda Song, Yifei Zhou, J. Andrew Bagnell, Akshay Krishnamurthy, Wen Sun (ICLR 2023).
-
SGD: The role of Implicit Regularization, Batch-size and Multiple Epochs,
with Satyen Kale and Karthik Sridharan (NeurIPS 2021). -
From Gradient Flow on Population Loss to Learning with Stochastic Gradient Descent,
with Satyen Kale, Jason D. Lee, Chris De Sa and Karthik Sridharan (NeurIPS 2022). -
The Complexity of Making the Gradient Small in Stochastic Convex Optimization,
with Dylan Foster, Ohad Shamir, Nathan Srebro, Karthik Sridharan and Blake Woodworth (COLT 2019, Best Student Paper Award).
Recent News
- [Apr'24] New paper on arXiv on efficient Agnostic RL under reset access to the environment. Accepted to COLT 2025.
- [Jan'24] New paper on arXiv on practical watermarking of Large Language Models (LLMs). Accepted to ICML 2025.
- [Jan'24] Our paper on deterministic linear bellman complete MDPs accepted at ICLR 2025 as Oral Presentation.
- [Jan'24] Our paper on evaluating machine unlearning algorithms accepted at ICLR 2025.
- [Dec'24] I am attending NeurIPS 2024. Let us meet if you are around?
- [Dec'24] Giving a short talk at the Princeton Language and Intelligence seminar on "Machine Unlearning: Where does the practice stand?".
- [Dec'24] Giving a talk at Rutgers University on learning-unlearning schemes.
- I will be an area chair for Algorithmic Learning Theory (ALT) 2025.
Selected Awards
- Best Student Paper Award at COLT 2019
- Finalist for Meta AI PhD Fellowship, 2022
- Best Talk Award, Honorable Mention, New York Academy of Sciences, 2020
- President's Gold Medal, IIT Kanpur, 2016
Publications and Preprints
In keeping with the convention in mathematical sciences, most of my papers list authors alphabetically by their last names. These papers are marked with the symbol "@" above my name. Otherwise, * denotes equal contributions.
Invited Talks
Invited Talks
Teaching
Teaching Assistant at Cornell University
-
Machine Learning Theory - Fall 2018 (Graduate Level Course)
Under Prof. Karthik Sridharan, Cornell University -
Introduction to Analysis of Algorithms - Spring 2018
Under Prof. Robert Kleinberg, Cornell University -
Machine Learning for Data Science - Fall 2017
Under Prof. Karthik Sridharan, Cornell University
Teaching Assistant at IIT Kanpur
-
Fundamentals of Computing - Fall 2015
Under Prof. Nitin Saxena, IIT Kanpur
Misc
How to reach me?
Please feel free to reach out if you think that we have any shared interests. The best way to reach me is via email at sekhari [at] mit [dot] edu.
Useful Links
- "You and Your Research", Richard Hamming (June 6, 1995).
- "How to Have a Bad Career in Research/Academia", a talk by David Patterson.
- "Last Lecture: Achieving Your Childhood Dreams", a talk by Randy Pausch at CMU.
- Witty Quotes by J. Michael Steele.
- Arxiv Latex Cleaner.
Personal Interests
Outside my research activies, I find joy in indoor climbing, experimenting with plant-based cooking, and consuming an absurd amount of chocolate.
A Fascinating Art
In 1989, Keith Haring created a print series titled The Story of Red and Blue, where he depicted characters inspired by both well-known children's stories and more mysterious sources. Throughout the series, the red and blue hues alternate, eventually merging within a human hand, symbolizing unity and conveying a message of hope and optimism. I find this artwork incredibly captivating and hope you enjoy it as well.
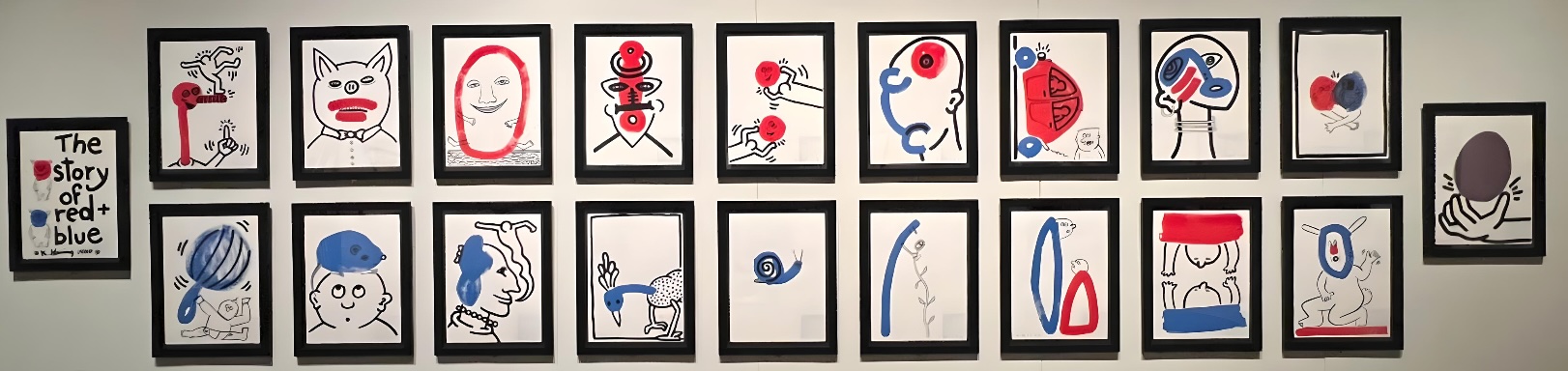
Professional Service
Learning Theory Alliance
Our mission is to develop a strong, supportive learning theory community and ensure its healthy growth by fostering inclusive community engagement and encouraging active contributions from researchers at all stages of their careers.
Please feel free to contact us for any questions / feedback / suggesions regarding LetAll. We would love to hear from you.Other Professional Activities
- Organization
- Organized workshop on Updatable Machine Learning at ICML 2022 (with Prof. Jayadev Acharya and Prof. Gautam Kamath)
- Poster sessions committee at Women in Data Science, Cambridge 2024.
- Communications Committee at Learning Theory Alliance (2023-current).
- Organization of Mentoring Tables at Fall 2023 Mentoriship Workshop under Learning Theory Alliance.
PC Member
- Algorithmic Learning Theory (ALT) 2024
- Conference on Learning Theory (COLT) 2019-22 (Junior PC Member)
Reviewing
- Journals: Journal of Complexity 2021, JMLR (2020-22)
- Conferences: NeurIPS (2019-22), ALT (2020-22), ICLR 2019, AISTATS 2019, ICML (2019-21), ISIT 2020, ITCS 2020, FORC 2021
Contact
The best way to reach me is via email.
sekhari [at] mit [dot] edu
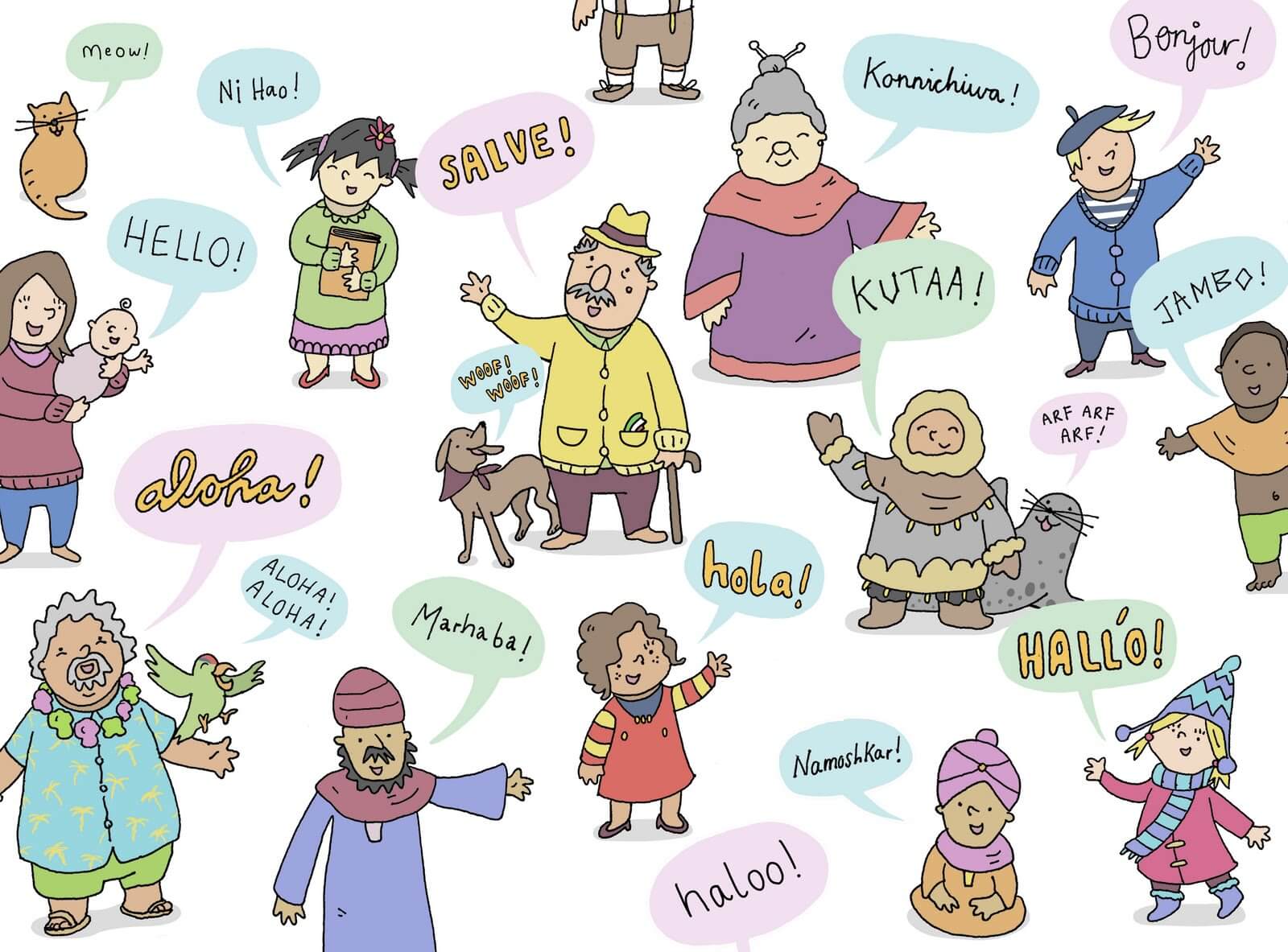